Multi-frequency MEMS Acoustic Emission Sensor (NSF CAREER) Heading link
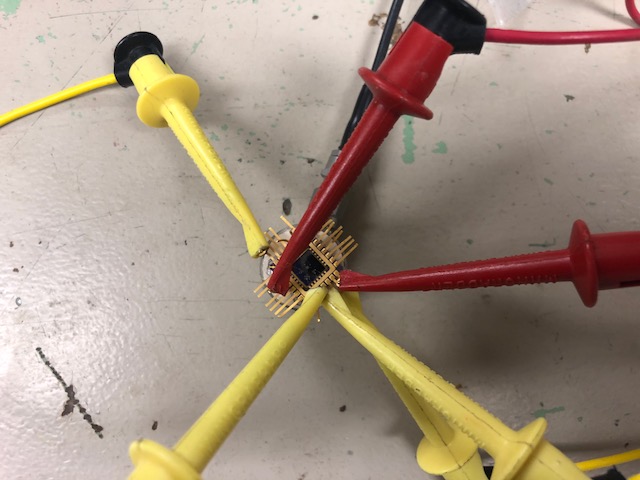
MEMS sensors have advantages of light weight and low cost as compared to conventional bulky sensors. They can be mass manufactured rapidly and reliably. The acoustic stability and quality of piezoelectric thin films increase their role in MEMS device manufacturing. Piezoelectric MEMS resonators have been developed sensing variety of input signals such as wine fermentation, density and viscosity sensing in liquids, dew point temperature. The output principle is based on monitoring changes in resonant behavior of sensor with respect to liquid properties.
In this study, piezoelectric MEMS resonators are considered to sense mechanical vibration generated in solids by crack surface movement, known as acoustic emission (AE). Piezoelectric MEMS resonators have limited sensitivity and bandwidth as compared to conventional bulky piezoelectric AE sensors. To break this limitation, multiple highly resonant MEMS piezoelectric sensors are connected in an array such that the total response is amplified by the constructive interference of carefully selected frequencies.
Noise blocking with Acoustic Metamaterials (NSF CAREER) Heading link
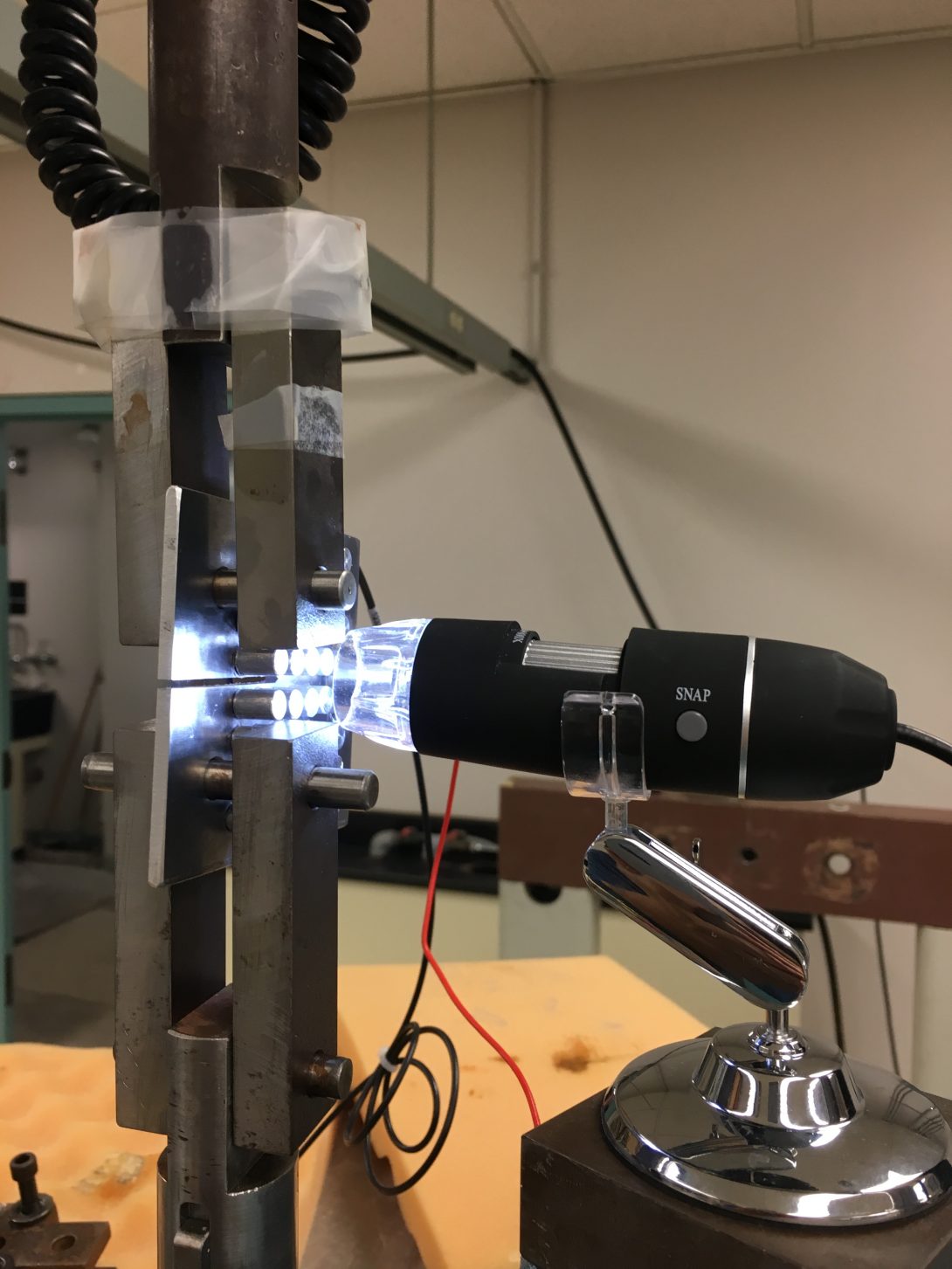
Among many NDE/SHM techniques, acoustic emission (AE) method provides continuous real-time data from active defects in structures. The AE method has been utilized to detect fatigue crack in metallic structures since 1970s. The AE method is based on detecting elastic waves released by sudden stress–strain changes within a material. The AE method has been applied for real-time damage detection, localization and characterization of fatigue crack in metallic structures. In a typical bridge application, minimum three sensors are required to monitor the localized crack activity such that two-dimensional localization can be achieved, and the influence of secondary emissions are minimized.
In this study, friction emissions are blocked by applying elastic metamaterial filters in the path between friction emissions and AE sensor such that single AE sensor is sufficient to effectively detect the fatigue crack growth.
Noise isolation to monitor a particular location in plate-like structures is developed using two-dimensional phononic crystals (PCs) such that a single AE sensor is sufficient to monitor the crack activity. PCs, also known as acoustic metamaterials, are artificial composite, man-made periodic systems creating new responses through physical constraints in the constituent materials.
We demonstrated that we minimize the detection of friction emissions and the data set represents the primary emissions due to crack growth. As the data rate is reduced into a level such that in situ data processing and wireless transmission becomes possible.
Effective Distribution of Bolt Holes in Gusset Plates for Early State Damage Detection (NSF CAREER) Heading link
Bolted connections in steel truss bridges form the weakest link due to higher risk of defect development due to stress corrosion cracking or galvanic corrosion between the connected elements. In conventional practice, bolts are placed based on minimum and maximum spacing requirements guided by AISC. In this study, the detectability of defects is added as a design variable when the bolts are placed. The periodic arrangement of bolts introduces unique frequency response that can be utilized in the evaluation of gusset plates based on the hypothesis that the periodic response is influenced by the presence of crack or the section loss.
Focusing Elastic Waves in Pipe-like Structures using GRIN Lens (NSF CMMI 1914663) Heading link
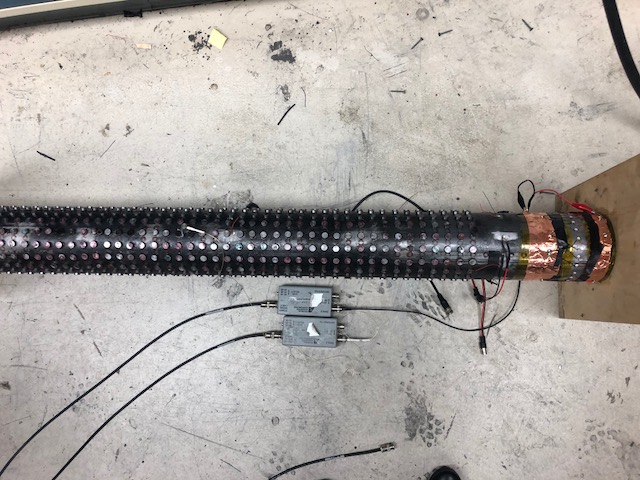
Metamaterials and phononic crystal (PC) structures are of growing interest due to their ability to manipulate and control the propagation of elastic waves in ways that are not possible in conventional materials. They are artificially engineered periodic structures composed of locally resonant unit cells or PCs. Due to their unique properties such as band gaps and the ability to slow the velocity of waves, metamaterials/PC structures offer the potential for a variety of applications including vibration and noise attenuation, frequency filtering, cloaking, sensing, and refractive acoustic devices among others.
We explore a novel conformal Gradient-Index Phononic Crystal (GRIN-PC) lens integrated within a pipe to amplify ultrasonic wave modes used in guided wave testing of pipelines toward enhanced sensing and improved damage detection. These refractive elastic metastructures can be created by engineering the dispersion curves to focus wave energy which can also benefit many applications such as energy harvesting, sensing and subwavelength imaging. The proposed conformal lens is composed of an array of cylindrical steel stubs attached to outer surface of the steel pipe which are tailored according to the hyperbolic secant profile of refractive index around the circumference of direction to focus the guided wave energy transmitted by ultrasonic wave in axial direction.
Real-time Weld Monitoring using Acoustic Emission and Ultrasonics (DMDII, current MxD) Heading link
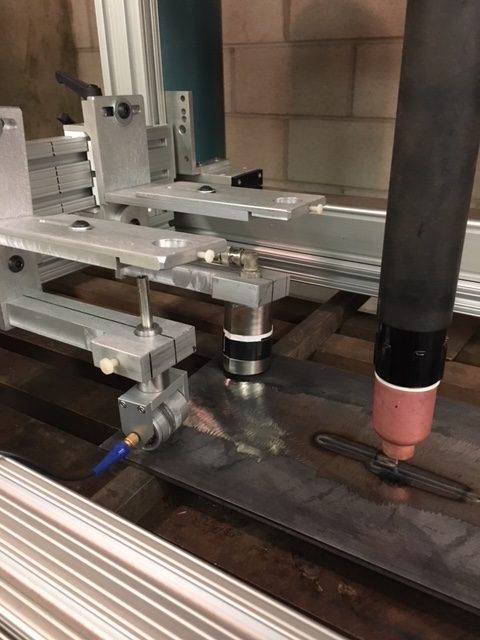
The problem is tackled by integrating multiple sensor outputs into a machine learning algorithm. With proper real-time weld monitoring methods, weld defects are expected to be recognized, and the welder can correct the weld parameter immediately. Whether in a manual or an automated weld process, the experience of the welder is a decisive factor. In addition, it is difficult for the welder to make a rapid and appropriate decision with a high number of parameters from the welding machine and monitoring system. Meanwhile, with a manual decision involved, the automaticity of the weld is limited. With the aim to solve this problem, intelligent decisions in response to process and monitoring variables offer a great potential solution. Through building a training set, machine learning algorithms can analyze the data—including weld parameters and monitoring variables during the weld process—assess the weld quality, and give a reasonable assessment. By keeping collected data and improving the training set during the weld, the accuracy of the machine learning algorithm can subsequently be improved over time.
The automated weld quality assurance can improve efficiency and productivity. In this study, real-time weld quality assurance approach using acoustic emission (AE) and air-coupled ultrasonic testing (UT) is studied. The in-situ monitoring system evaluates the changes in weld size leading to burn through by changing the weld heat input. While UT method provides quantitative information about the weld state, any localized surface discontinuity causes sudden surges in the AE energy indicating nonuniform welding qualitatively. Passive and active nondestructive evaluation methods should be combined to monitor weld quality real time for qualitative and quantitative assessment.
The sensor data utilized in the machine learning algorithm include acoustic emission (hit driven and time driven) and welding data (gas flow rate, current and voltage inputs). The acoustic emission (AE) associated with weld parameter monitoring is chosen as the real-time monitoring methods for automated welding. That is because a variety of acoustic activities are generated within the welding process. The algorithm is evaluated using data recorded at three laboratories in UIC, ITW Miller, and John Deere. Multiple machine learning algorithms are tested.
Microstructure Characterization by Means of Nonlinear Ultrasonics and Acoustic Microscope (NSF CMMI 1463501 and ONR) Heading link
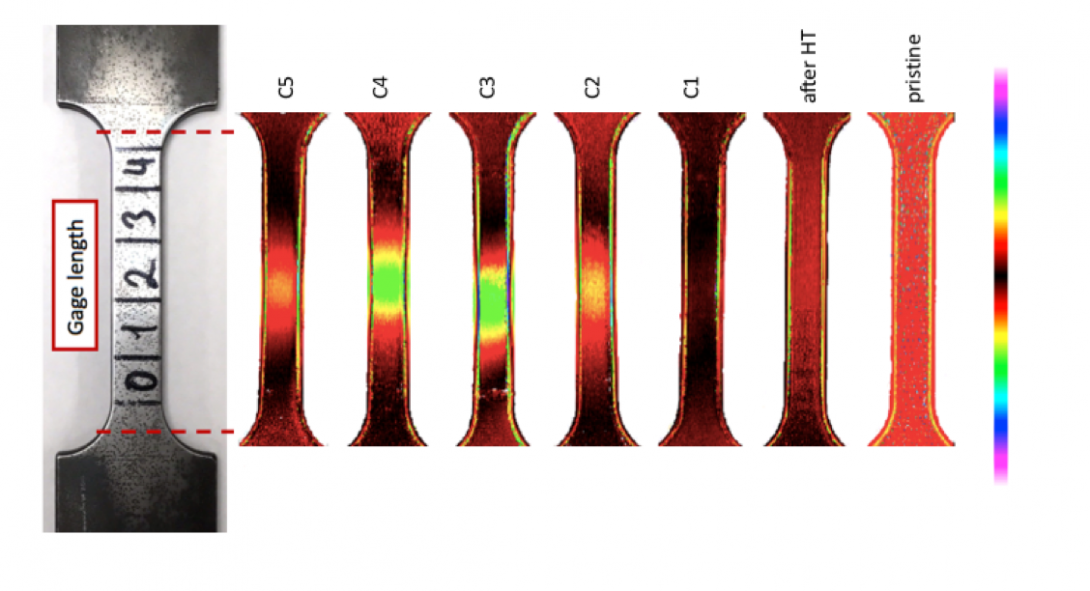
In this study, the creep damage in stainless steel 410 specimens are assessed in the immersed nonlinear ultrasonics experimental setup by integrating third harmonic generation method and the Superlattice (one dimensional metamaterial). Superlattice is used with the purpose of blocking the non-linearity generated in water and instruments. It is expected that the calculated non-linearity in the received signal consists of only the non-linearity of micro-structural features in solid. Consequently, the sensitivity of the nonlinear ultrasonics to detect the micro-structural changes in materials is improved.